Introduction
The cybersecurity landscape is evolving rapidly, with threats becoming more sophisticated and frequent. Traditional techniques of defense, which once sufficed, are now inadequate against the complex cyber-attacks we face today. In response, Artificial Intelligence (AI) has emerged as a game-changer. AI-driven cybersecurity solutions are transforming threat detection, making it more proactive, efficient, and precise. This article explores how AI is reshaping the cybersecurity field in 2024, delving into the technologies and methodologies that are driving this change.
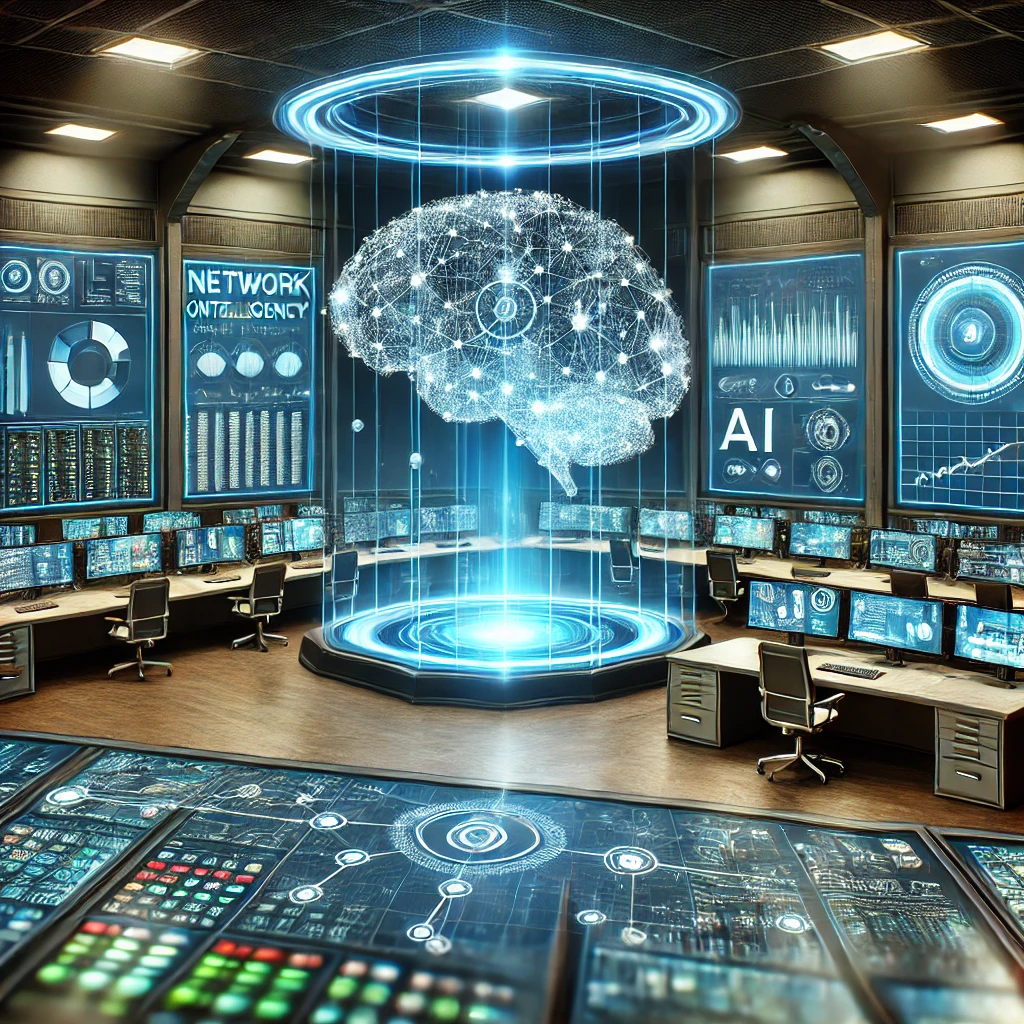
The Shift from Reactive to Proactive Security
Traditional Threat Detection Limitations
Historically, cybersecurity relied heavily on reactive measures. Signature-based detection, where known threat signatures are matched against incoming data, was the standard. Nonetheless, this approach has significant limitations. It cannot detect new, unknown threats, leaving systems vulnerable to zero-day attacks. Moreover, the increasing volume and complexity of threats have outpaced the capabilities of traditional security tools.
AI’s Proactive Approach
In 2024, AI is enabling a shift towards proactive threat detection. Unlike traditional approaches, AI doesn’t rely solely on known signatures. Instead, it uses machine learning (ML) algorithms to analyze patterns in data and detect anomalies. This allows AI to detect potential threats before they can cause harm. By constantly learning from new data, AI systems can adapt to evolving threats, providing a dynamic defense mechanism.
Machine Learning at the Core of AI-Driven Security
Supervised and Unsupervised Learning
Machine learning is the backbone of AI-driven cybersecurity. It encompasses various techniques that allow systems to learn from data. Supervised learning involves training models on labeled data, where outcomes are known. This method is effective in environments where specific threats are well-documented. On the other hand, unsupervised learning identifies patterns in data without prior knowledge of the outcome. This approach is particularly useful for detecting unknown threats. The system learns to recognize what “normal” looks like and flags deviations from this norm.
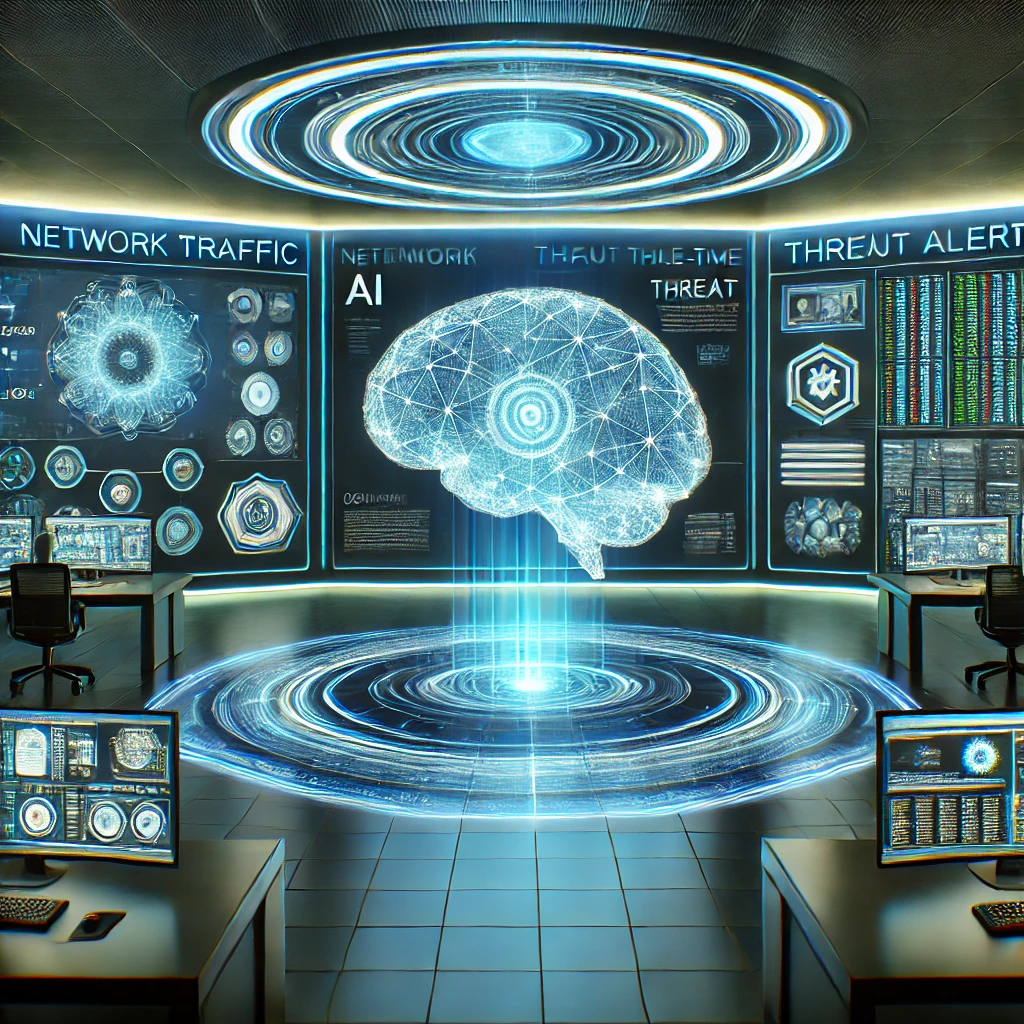
Reinforcement Learning for Continuous Improvement
Another key ML technique is reinforcement learning. Here, AI systems learn by receiving feedback on their actions. This approach is ideal for developing autonomous threat response systems. The AI can adapt to new threats without human intervention. Over time, the system becomes more efficient, reducing the need for manual oversight.
Deep Learning and Its Impact on Cybersecurity
Advanced Pattern Recognition
Deep learning, a subset of machine learning, has revolutionized pattern recognition. It uses neural networks with multiple layers to process and analyze vast amounts of data. In cybersecurity, deep learning models are particularly effective at identifying complex patterns that traditional algorithms might miss. For example, convolutional neural networks (CNNs) excel in image recognition. They are now being used to analyze network traffic. They also detect anomalies.
Real-Time Threat Detection
In 2024, real-time threat detection is critical. Recurrent neural networks (RNNs), designed for sequence analysis, are highly effective in this area. They can observe user behavior, like login patterns, and detect when something deviates from the norm. This ability is essential for identifying insider threats or compromised accounts before they can cause significant damage.
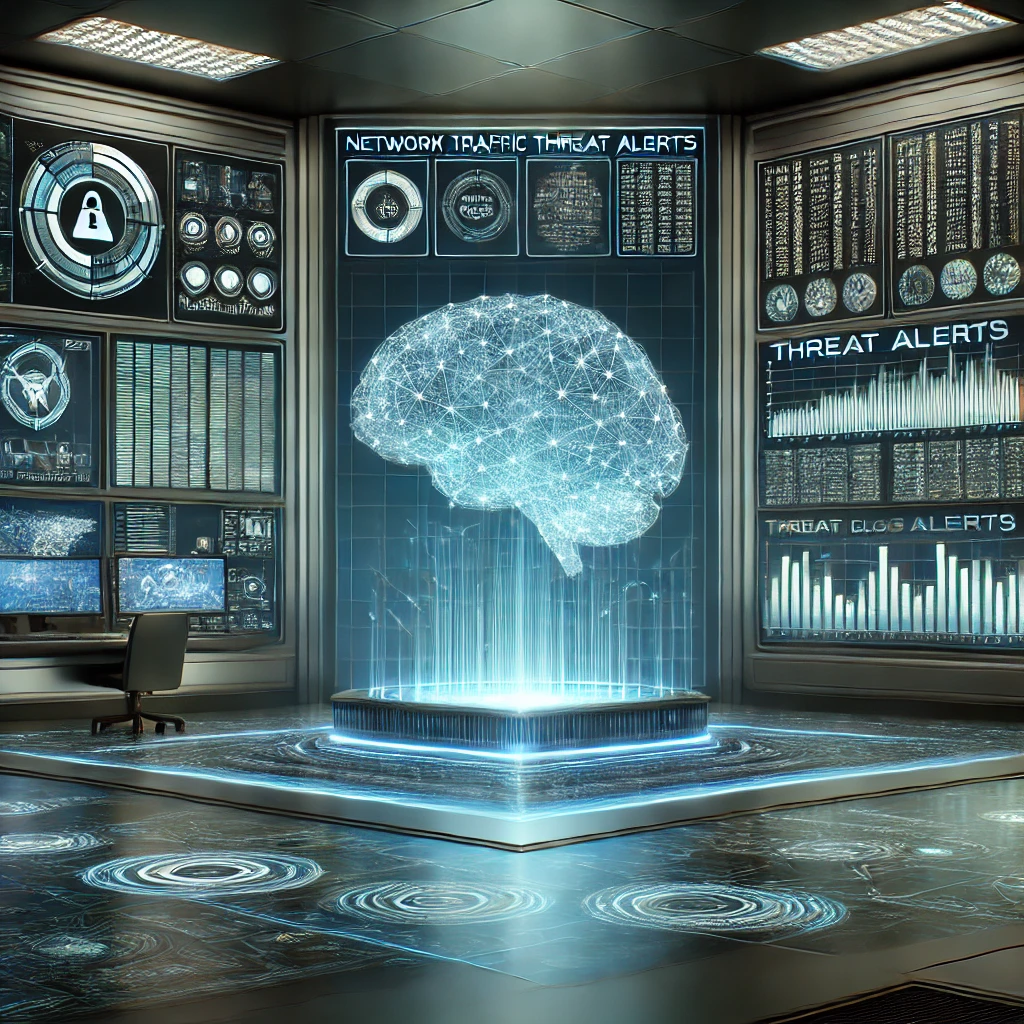
Autonomous Threat Response: The Future of Cyber Defense
The Rise of Automated Defense Systems
One of the most significant advancements in AI-driven cybersecurity is autonomous threat response. These systems detect, analyze, and respond to threats with minimal human intervention. In 2024, Security Orchestration, Automation, and Response (SOAR) platforms are increasingly incorporating AI to enhance their capabilities. These platforms can automate routine security tasks. They can isolate infected systems or block malicious IP addresses. This frees up human analysts to focus on more complex issues.
Case Study: Darktrace’s Autonomous Response
Darktrace, a leader in AI-driven cybersecurity, has pioneered the use of autonomous response technologies. Their AI system is known as the Enterprise Immune System. It mimics the human immune system by learning what is normal for a network. It responds to deviations. In one notable case, Darktrace’s AI detected unusual behavior on a client’s network, indicative of a ransomware attack. The AI automatically took action to contain the threat, preventing it from spreading and causing significant damage.
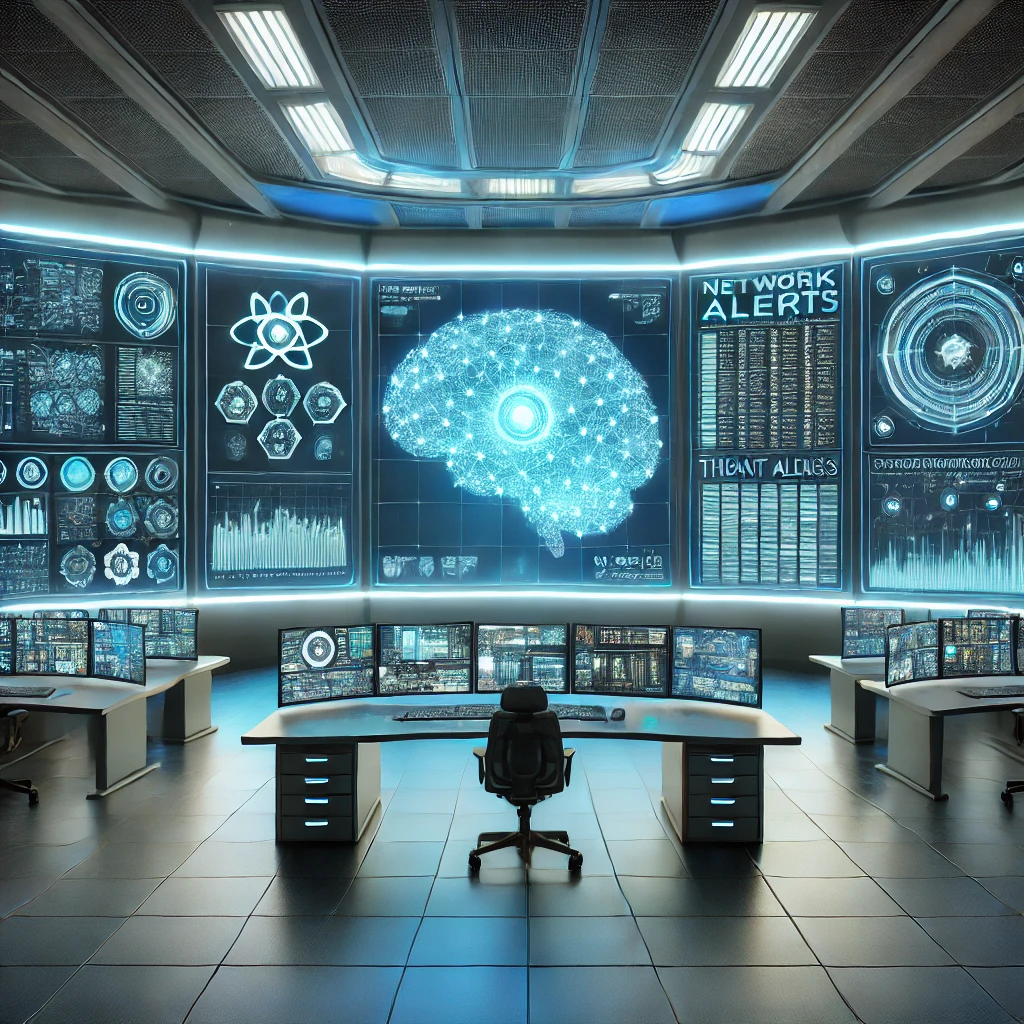
Natural Language Processing in Cybersecurity
Enhancing Phishing Detection
Phishing remains a top concern for organizations, as attackers increasingly use sophisticated techniques to deceive users. Natural Language Processing (NLP) has become a crucial tool in combating phishing. NLP algorithms analyze the content and context of emails, looking for patterns that show phishing attempts. In 2024, AI-powered phishing detection tools can find even the most subtle signs of a phishing try. These tools adapt quickly to new tactics used by cybercriminals.
Threat Intelligence and NLP
NLP also plays a vital role in threat intelligence. NLP algorithms can identify emerging threats by analyzing vast amounts of unstructured data. This includes social media posts, dark web forums, and threat reports. These algorithms also give actionable insights. This allows cybersecurity teams to stay ahead of attackers by anticipating their moves and implementing preemptive measures.
Challenges and Ethical Considerations
Addressing AI Bias
Despite its many advantages, AI-driven cybersecurity is not without challenges. One significant concern is the potential for bias in AI algorithms. If an AI system is trained on biased data, it may make incorrect decisions. For example, it might flag legitimate activities as suspicious. This can lead to a high number of false positives, which can overwhelm security teams and reduce overall efficiency. Hence, it is crucial to ensure that AI systems are trained on diverse datasets and continuously monitored for bias.
Ensuring Regulatory Compliance
Another challenge is ensuring that AI-driven cybersecurity solutions comply with regulatory requirements. Data privacy regulations, such as GDPR and CCPA, place strict controls on how personal data is collected, processed, and stored. Organizations must ensure that their AI systems do not violate these regulations, especially when it comes to automated decision-making processes. Transparency in AI decision-making and regular audits can help address these concerns.
The Future of AI-Driven Cybersecurity
Integration with Quantum Computing
As AI continues to evolve, its integration with other emerging technologies will further enhance cybersecurity. Quantum computing, for example, has the potential to significantly increase the processing power of AI systems. This could enable faster and more accurate threat detection. AI algorithms would be able to analyze even larger datasets in real-time. While still in its early stages, the combination of AI and quantum computing could revolutionize cybersecurity in the coming years.
The Role of Human Expertise
Despite the advancements in AI, human expertise remains indispensable in cybersecurity. AI systems are highly effective at automating routine tasks and analyzing vast amounts of data. However, human analysts are still needed to interpret the broader context and make strategic decisions. The future of cybersecurity will likely involve a hybrid approach. AI and human expertise will work together. This collaboration will provide a comprehensive defense against cyber threats.
Conclusion
AI-driven cybersecurity is transforming the way organizations detect and respond to threats in 2024. By leveraging machine learning, deep learning, and NLP, these technologies offer a proactive and dynamic approach to cybersecurity. However, it is essential to address the challenges associated with AI. These include bias and regulatory compliance. Addressing these challenges is crucial to ensure that these systems are used effectively and ethically. As we look to the future, the integration of AI with emerging technologies promises to take cybersecurity to new heights. Quantum computing will provide even more robust defenses. These defenses will be against the ever-evolving landscape of cyber threats.